ポスタータイトル
SSVD Based Biclustering Methods via Mixed Prenet Penalty
発表者
Jiqiang Wang and Kei Hirose
Abstract:
Standard clustering methods typically group samples based on their entire set of observed features. In large datasets, however, only a few features may play a role in distinguishing different clusters.
In our research, we observed that if certain biclusters produced by the algorithm are excessively similar, which means they have a high degree of repetition (overlap). Such redundancy can pose challenges to our analysis because it is difficult to identify the useful variables. On the other hand, these repeated portions may also contain valuable information. However, a simple prohibition or allowance of repetition is not sufficient. We need to find a method to identify when it is necessary to retain duplicated parts. In our study, we successfully improved the SSVD (Sparse Singular Value Decomposition) by proposing the following mixed Prenet penalty (a hybrid of Prenet (product-based elastic net) penalty and the original elastic net penalty) to replace the original adaptive Lasso penalty in the SSVD method.
The Prenet penalty was originally proposed by Hirose and Terada (2022) to deal with the loading matrix in Factor analysis. It is based on the product of a pair of elements in each row of the loading matrix. The Prenet not only shrinks some of the factor loadings toward exactly zero but also enhances the simplicity of the loading matrix, which plays an important role in the interpretation of the common factors. However, the original Prenet penalty itself cannot provide a good clustering result in our experience, then we extended it to make it compatible with the general elastic net and allow users to easily control the threshold for allowing overlapping by adjusting parameter values. This improvement has a noticeable effect on reducing the degree of dummy overlapping.
受賞日
2023年12月8日
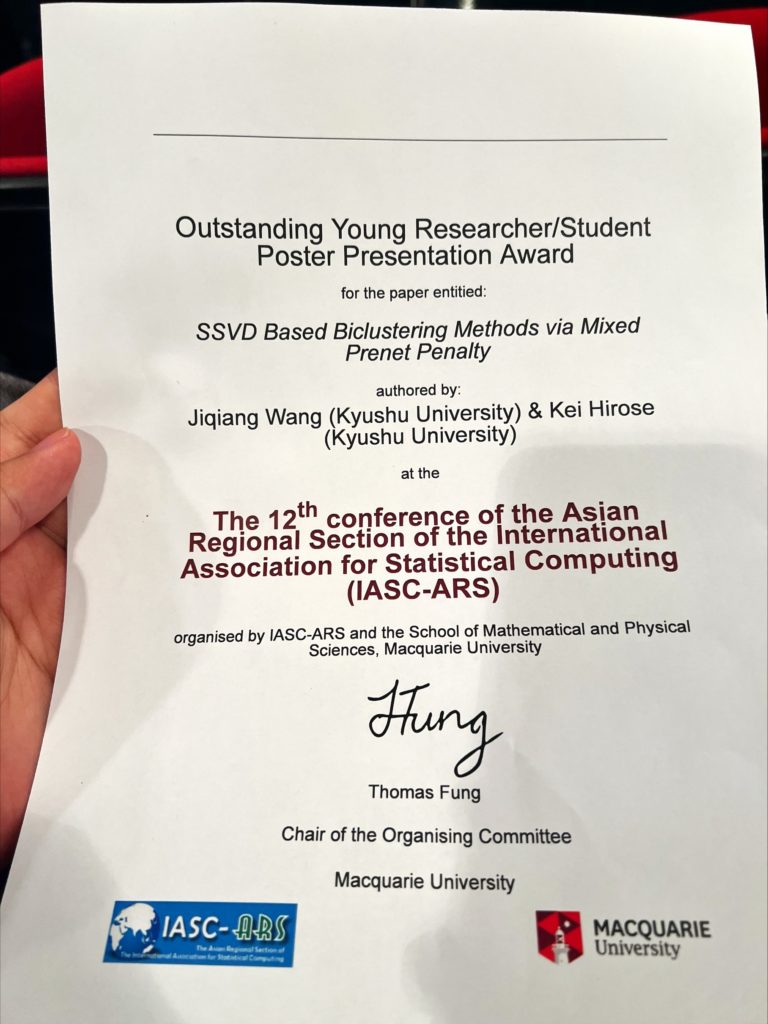